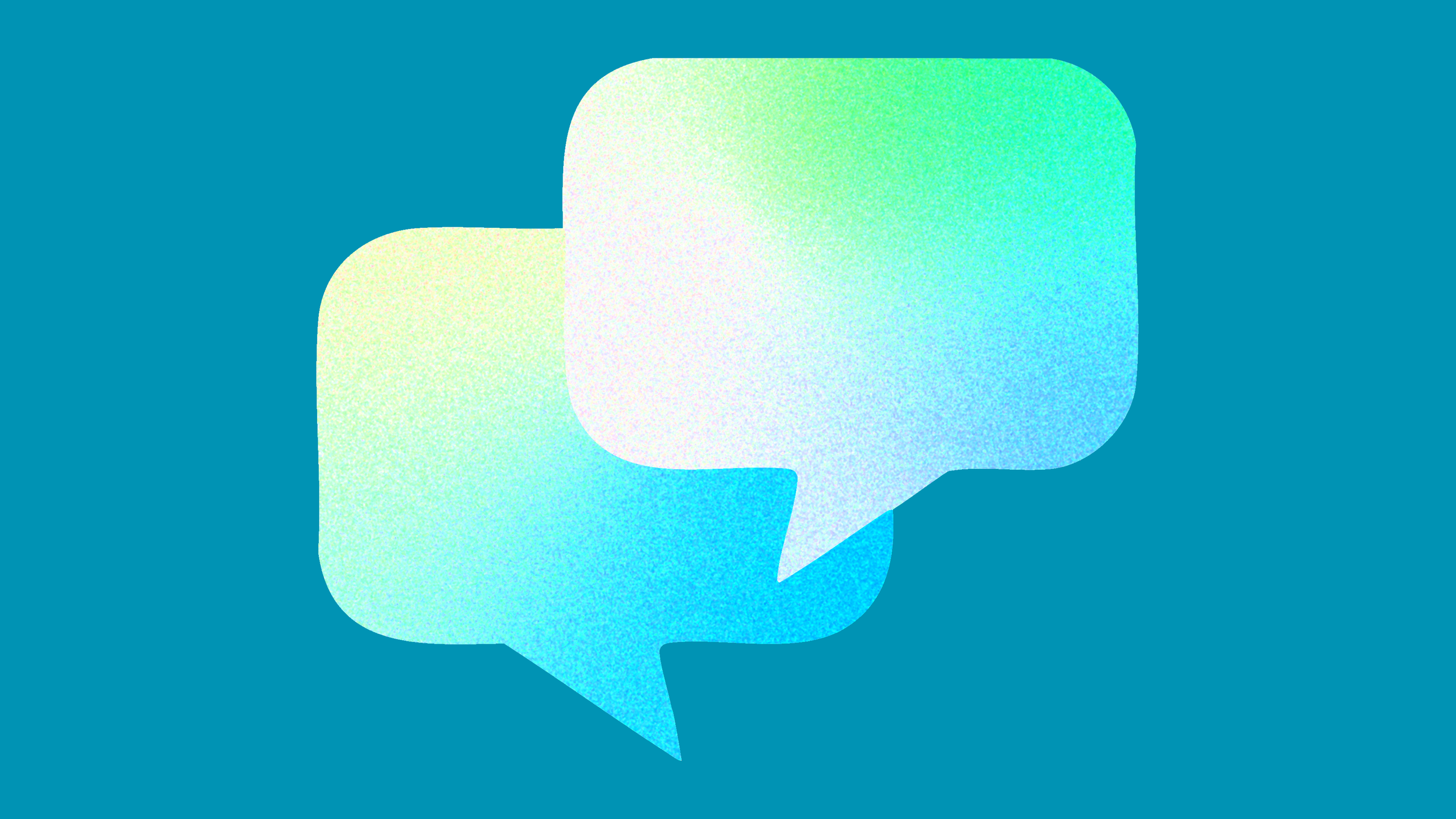
Meet the Team: Corbin Foucart, Deep Learning Researcher
CLIKA’s mission is to connect AI software to various hardware around us. CLIKA's AI compression technique, developed in-house, dramatically reduces the size of AI models without sacrificing their performance, enabling businesses to quickly, economically, and reliably commercialize their AI applications on devices used in our daily lives.
In this blog, we will be interviewing Corbin Foucart, a deep learning researcher at CLIKA. His role at the company is to take part in engineering projects with Ben Asaf, the Chief Technology Officer (CTO), along with two other DL engineers on our team toward building CLIKA’s auto TinyAI toolkit.
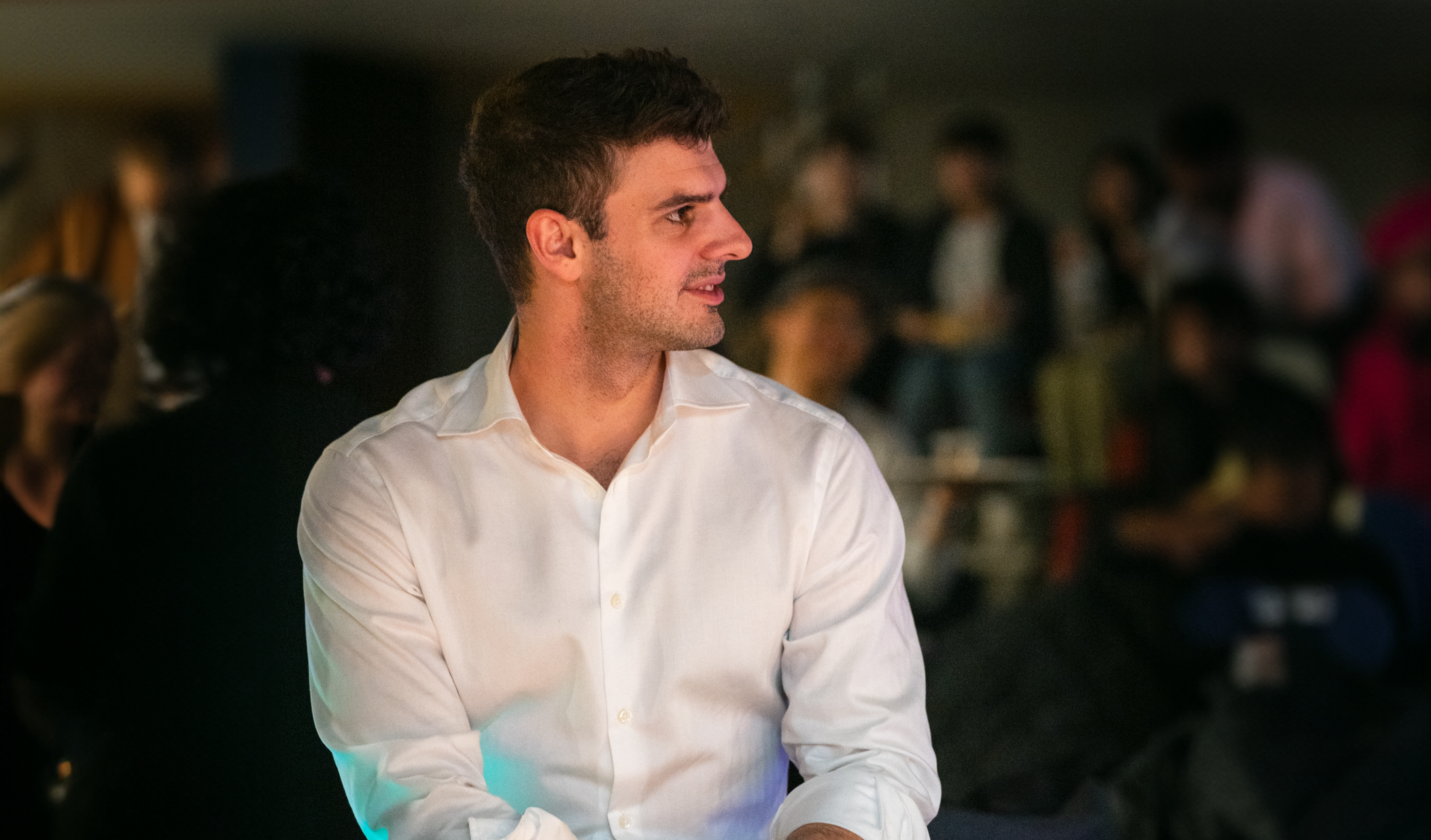
Prior to joining CLIKA, Corbin was completing his PhD at the Massachusetts Institute of Technology (MIT) in Computational Science and Engineering. Although Corbin was born in California, he has lived both abroad and in numerous places growing up. He is an applied math and physics enthusiast, starting from his undergrad days at Stanford University.
Q. Can you tell us about your personal background?
I was born in the San Francisco Bay Area in California, right outside of Berkeley. I moved around a lot growing up and I’ve lived in more than 15 places so far, with my top three being Boston, Berlin, and Hong Kong. I’m particularly drawn to the innovative tech ecosystem in the Asia-Pacific (APAC) region because I find it has this crazy fusion of the old and the new. Rapid industrialization has resulted in super technologically advanced cities like Seoul and Tokyo, but you can also find regions where people live in pre-industrial cities but have smartphones in their hand. I think this unique environment contributes to an innovation hotbed with opportunities and challenges that don’t exist in Western markets.
Q. Why did you get into engineering?
I’m generally interested in applying ideas from math to model and analyze complicated processes that arise in the real world—so physics, statistics, and computer science have always piqued my interest. Growing up, I knew that when I got to college, I wanted to do something related to modeling and understanding the world, but I didn’t really know what that entailed at the time. So at the end of high school I applied to universities based on how good their physics programs were, since I knew I liked solving physics problems. I ended up choosing Stanford because its physics department was good, but also because the campus was warm and sunny—four years of high school in New England had really made me miss seeing palm trees.
But then when sophomore year came around, I realized that studying physics theory by itself wasn’t super satisfying to me because it was very abstract. If you work in physics theory, the typical life path is to become a professor and lay out conjectures as to how the universe behaves. Of course, that type of work is beautiful and important, but it might take an entire lifetime before your conjectures are substantiated. Your work may be hugely impactful but take years to reach any practical application; or worse, you might work on a branch of theory for years that turns out to be wrong or inconclusive.
For example, in the 1960s, Higgs proposed a mechanism to explain why the most basic building blocks of the universe have mass. But only recently was it materially substantiated, about 50 years later. Perhaps in the future, these advances in theory will enable new advances in engineering as well, but the trickle-down from theory to practice is typically a slow one. Because of this lag, studying pure physics often felt like I was looking at a map of a place where I was never going to go.
By the end of my undergrad, I had become much more interested in computing and applied math. I worked at the Weierstrass Institute, which is a research institute in Germany for applied math and stochastic analysis, where I became interested in computational physics. The main approach in computational physics is to describe a real-world physical process with a complicated differential equation that you have no hope of solving with pen and paper. But even though you can’t write down a solution, you can discretize the equation on a computer and solve it to an arbitrary degree of accuracy. I thought that was awesome. Plus, as a nice side benefit, the turnaround time on knowing whether your answer is right is almost always less than 50 years, although it isn’t uncommon to run a simulation for a week or two!
I went to MIT for grad school to continue working on developing new methods in computational physics. The problems I was working on were in the field of fluid dynamics, a discipline which more-or-less revolves around one set of equations—the Navier-Stokes equations—that govern how all fluids move, from the milk in your coffee all the way up to the formation of hurricanes over the ocean. Then towards the end of my PhD, I became really interested in machine learning (ML) techniques because they were taking off and smashing benchmarks in other fields but were achieving, to put it politely, limited success solving problems in computational physics. I became interested in developing ML models which could accelerate classical numerical solvers without replacing them, allowing the solutions to be computed in an efficient way but still arriving an answer within the rules of the governing equations. These days, people talk about so-called “hallucinations” in large language models—physics doesn’t hallucinate, and neither should physics-based models, ML-accelerated or otherwise.
Q. How did you find out about CLIKA?
I was actually in the process of choosing between competing offers for quantitative finance roles when I stumbled upon CLIKA’s LinkedIn posting. What drew me to CLIKA was the founders’ pitch as well as their mission to make AI lightweight, which struck me as very well-timed, given the explosion of large models in the past few years. The recent trend in AI has been a one-track story of models getting bigger, bigger, and bigger. And it’s great—we're squeezing a ton of performance out of them and doing things that would have seemed impossible merely few years ago, but after the honeymoon period, there will come the inevitable hangover questions:
- How do we democratize these models? And "democratize” isn’t just a buzzword; a world where only a few, gigantic tech companies have sole access to these game-changing technologies could be a very scary one.
- How do we run them on everyday devices?
- How do we deploy them painlessly and efficiently?
I see lightweight AI as the key to addressing these questions.
Q. If you had chosen one of the finance roles, what would you have been doing?
I would have worked as a quantitative researcher. These researchers use a wide variety of techniques in computing and statistical inference to find market inefficiencies. It’s a challenging and fascinating field that I may return to someday.
Q. What are you currently doing at CLIKA?
As a deep learning researcher, I primarily investigate ways to make ML faster, more efficient and more lightweight. Sometimes, that’s integrating a new technology that comes from academia, or capitalizing on a well-engineered open-source solution from industry. And sometimes, it means innovating something new here at CLIKA. My work involves reading a lot of research papers and coding up prototypes of things that we think might be interesting or promising. From there, I experiment and iterate on the engineering design: if something shows promise, I’ll work with the deep learning team to bring it into production.
I think it’s the best job in the world because I get to play around with cutting-edge ideas in the AI revolution as it unfolds around us.
Q. What kind of people do you think will thrive at CLIKA?
We have a burning desire to know why things work, why things work well, and why things don’t work well. We approach our work scientifically and with a very skeptical mindset. We all come from very different backgrounds, but I think these characteristics are a unifying feature between us. I also think there’s this healthy mix of camaraderie and friendship among us that makes CLIKA a fun and inspiring place to work.
So people who relish fast prototyping, appreciate the close-knit-team vibe of a startup, and are eager to learn will excel here.
Q. What do you see yourself doing in the future at CLIKA?
It’s my hope that CLIKA will play an important part in the democratization of AI. It's going to be cool to see the direction that CLIKA takes as it scales.
As for me, I see myself continuing the work that I do right now. To use one of my favorite cliches, the problems that I am working on are kind of like fashion because they say that fashion is a never-ending job. Similarly, there’s always an imaginative way to squeeze more performance out of your machine, yielding faster and better models.
Personally, I get a huge thrill from creating improvements in speed and model performance, and with the never-ending demand for faster and more accurate models, I don’t see myself running out of interesting problems to solve anytime soon.